New AWS service lets customers rent Nvidia GPUs for quick AI projects
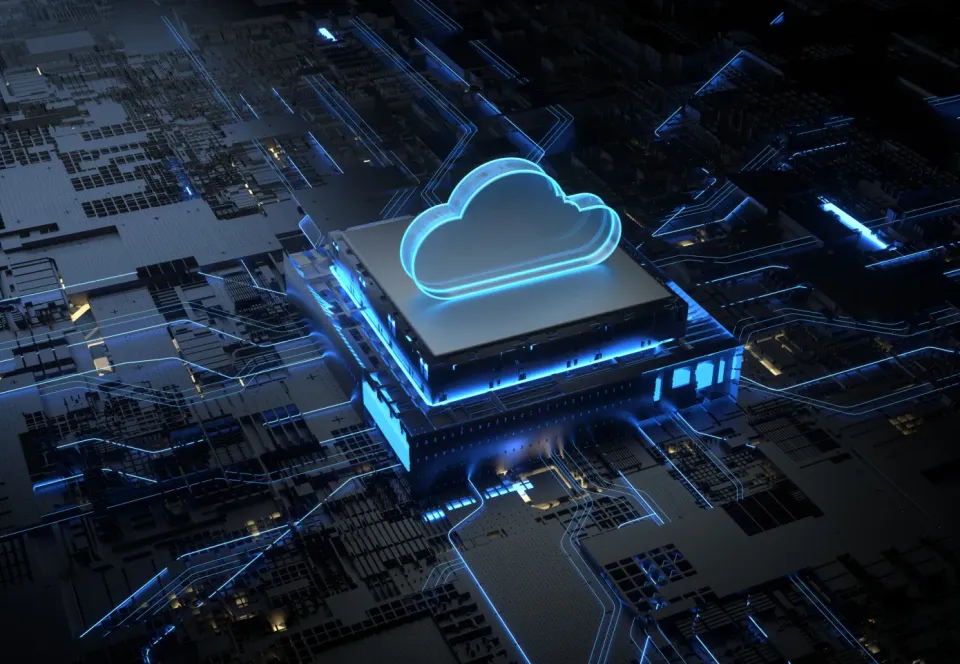
More and more companies are running large language models, which require access to GPUs. The most popular of those by far are from Nvidia, making them expensive and often in short supply. Renting a long-term instance from a cloud provider when you only need access to these costly resources for a single job doesn’t necessarily make sense.
To help solve that problem, AWS launched Amazon Elastic Compute Cloud (EC2) Capacity Blocks for ML today, enabling customers to buy access to these GPUs for a defined amount of time, typically to run some sort of AI-related job such as training a machine learning model or running an experiment with an existing model.
“This is an innovative new way to schedule GPU instances where you can reserve the number of instances you need for a future date for just the amount of time you require,” Channy Yun wrote in a blog post announcing the new feature.
The product gives customers access to Nvidia H100 Tensor Core GPUs instances in cluster sizes of one to 64 instances with 8 GPUs per instance. They can reserve time for up to 14 days in one-day increments, up to eight weeks in advance. When the time frame is over, the instances will shut down automatically.
The new product enables users to sign up for the number of instances they need for a defined block of time, just like reserving a hotel room for a certain number of days (as the company put it). From the customer’s perspective, they will know exactly how long the job will run, how many GPUs they’ll use and how much it will cost up front, giving them cost certainty.
For Amazon, they can put these in-demand resources to work in almost an auction kind of environment, assuring them of revenue (assuming the customers come, of course). The price for access to these resources will be truly dynamic, varying depending on supply and demand, according to the company.
As a users sign up for the service, its displays the total cost for the timeframe and resources. Users can dial that up or down, depending on their resource appetite and budgets before agreeing to buy.